Mitigating bias in voice AI modeling
March 7, 2023
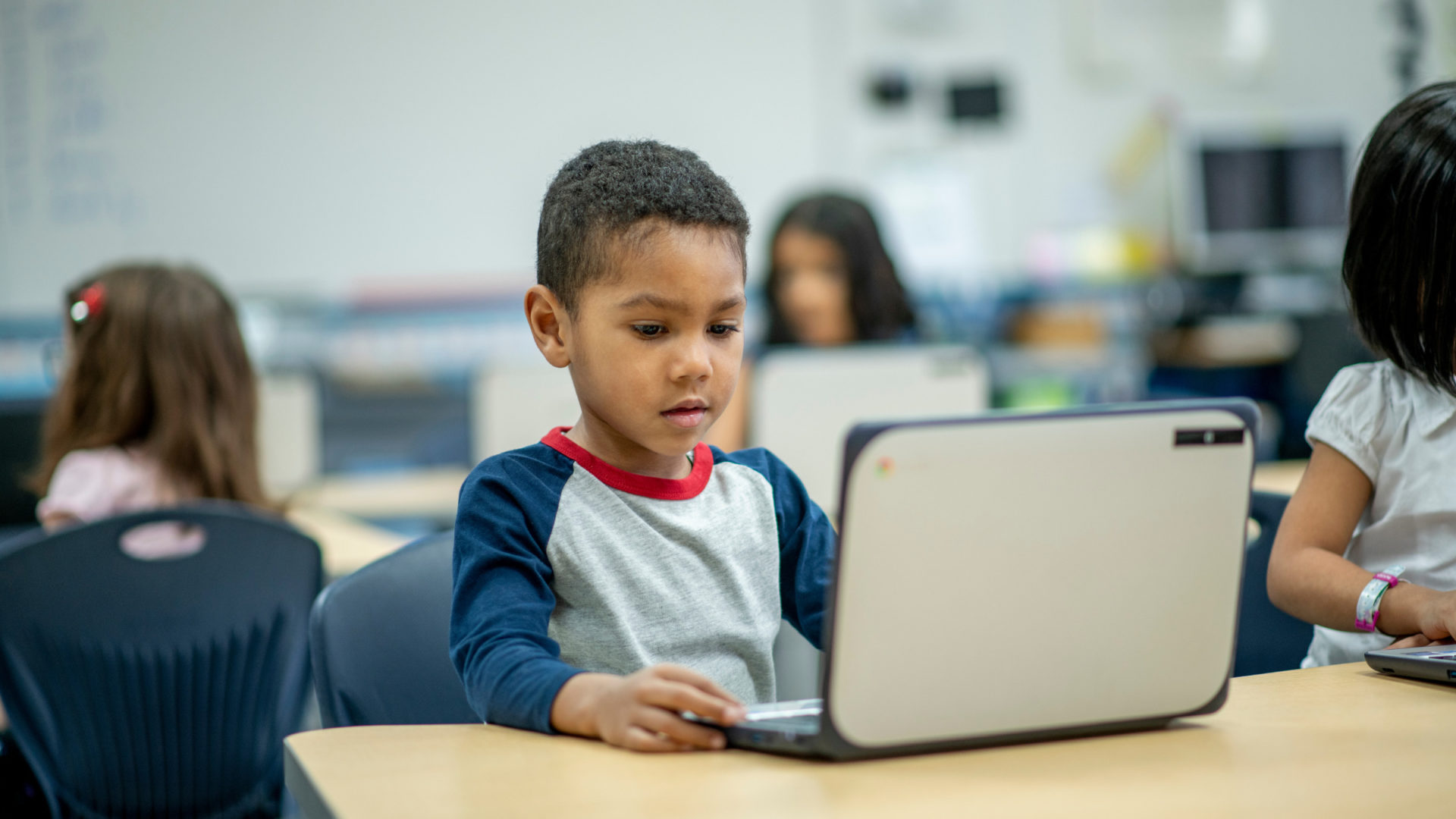
In an educational setting, voice AI systems must be highly accurate and understand all kids’ voices, regardless of their age, accent, dialect, or socioeconomic background to ensure equitable learning experiences.
Anything less, and the feedback children — and their teachers — receive can contain false negatives, which are frustrating, or false positives, which can dampen children’s confidence and negatively impact their learning journey.
For example, if a biased speech system fails to understand a child’s accent or dialect, it can consistently tell that child that they are a poor reader when, in fact, they are reading correctly.
An unbiased system, on the other hand, can offer fair and uncompromised information to facilitate edtech platforms and services.
SoapBox is an equity-by-design company, building voice AI technology that aims to understand every child’s voice accurately and equally, regardless of their race, background, age, or ethnicity.
In this blog, we explain how we proactively identify and mitigate racial bias in our data handling and modeling.
Generating a diverse data pool
Our training, development, and evaluation data sets are chosen from our in-house data pool, a proprietary data set of children’s speech audio.
In order to ensure diversity in any set deriving from this data pool, it must be as diverse as possible.
We take the following steps to maximize diversity in our data pool:
- Promote data collection efforts in as many countries as possible (193 countries)
- Promote data collection methods that maximize the number of speakers
- Enable data collection efforts on lower-end devices
- Target specific demographics for commissioning of training and test data
Mitigating bias in AI algorithms
The process of building and tuning AI models can introduce bias separate from the data used to train it.
We employ the following activities to improve our algorithms:
- Update models continuously by adding new data in fair and equitable proportions to our training set.
- Constantly update test sets, questioning their validity and whether they’re representative of the population.
- Employ rigorous testing – monitoring the TN, FN, TP, and FP and calculating metrics such as precision, recall, Cdet, f1 score, and word error rate (WER).
- Rebuild models weekly and re-run our evaluations to ensure no performance regressions over time.
- Specifically test model performance on different demographics.
Case study: Delivering equity for African American English
Example 1
We identified that if our dataset contains ONLY audio of Black students reading passages without any reading errors, we are underrepresenting those Black students who may be struggling. It is essential to know that our system works well for Black students at every level of reading ability.
Example 2
We identified that Black students who speak African American English will code-switch to General American English when asked to read a sentence written in the General American dialect.
Therefore, in order to test our system’s performance on African American English, we need to collect audio data of African American English speakers actually speaking their own dialect.
We designed a data gathering exercise (“Describe the Picture”) aimed at eliciting the natural speech that a student would use when speaking to their friends or family.
Our linguists conducted an in-depth linguistic analysis of African American English, and we took the following steps to update our model features based on this:
- Dictionary: We updated our dictionary to include African American English word pronunciations (that differed from our current entries by one or more phonemes).
- Training set: We identified data in our data pool that was labeled as African American English and added it to our training set for our acoustic model. We added lexical, syntactic, and morphological text examples to our language model training text.
- Test set: We built our test set of spoken audio data by African American English speakers.
Interested in learning more?
Here are some additional resources on mitigating bias in AI:
- Our conversation on AI, equity, and education with The EdTech Equity Project
- How SoapBox became the first company to earn the Prioritizing Racial Equity in AI Design certification from Digital Promise
- Reflections on designing equitable AI for education
If you’re ready to voice-enable your learning product for kids using our speech technology, Get Started now!